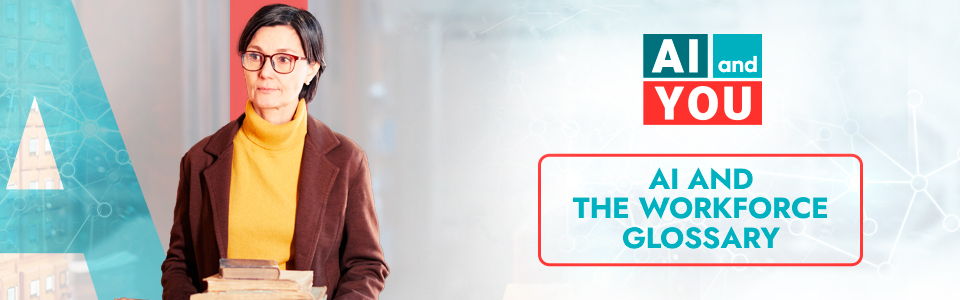
Job automation: Refers to machines and technology taking over tasks that people usually do. For example in the manufacturing industry, AI robots can do the repetitive tasks in an assembly line such as assembly, packaging, and quality control, that were otherwise completed by human workers.
Skill Biased Technology Change (SBTC): When new technologies, like AI, create more demand for some skills while making other skills less needed.
Augmented workforce: The concept of combining human labor with AI technologies to increase, or augment, productivity and efficiency in the workplace. A common example of where we will see this will be in customer service, where AI-powered chatbots will handle routine customer questions and human agents will work on more complex issues.
Digital Inequities: Inequities will be created when some populations adopt new AI tools while others do not due to lack of awareness. For example, only some small business owners will download an AI app on their device to manage inventory while others will miss the opportunity.
AI ethics and employment: Rules and guidelines for using AI in employment contexts, making sure it is fair, transparent, accountable, and respects privacy. For example, social media platforms collect large amounts of user data and personal information using AI. It is important to consider how social media companies collect, store, and use the data as well as consider consequences to user privacy.
Labor Market Polarization: The division of the labor market into high-skilled, high-paying jobs and low-skilled, low-paying jobs, with fewer opportunities for middle-skilled occupations. AI's impact on employment can contribute to this polarization.
Digital Skills Gap: Refers to the difference between the supply of workers who have digital skills, such as knowing how to use AI, and the demand for these workers by employers. Closing the digital skill gap is important for ensuring workers are prepared to work in AI-driven workplaces.
Routine jobs: Tasks in a job that are repetitive and follow a pattern. They don't need creativity or problem-solving. AI can often do these tasks because they're predictable. Examples include data entry, basic administrative tasks, and basic data analysis.